The data is collected by sensors, but it must then be analyzed and made to speak. To do this, we call on a data scientist. Let’s discover this job through the portrait of Dylan, datascientist at Heyliot!
Introduce yourself !
Hello, Dylan Lebreton, 24 years old, from a small town in Côtes d’Armor and currently juggling between Trémorel (the small town), Rennes where I am doing a work-study program at Heyliot and Toulouse where I am studying engineering. All this without addiction to coffee.
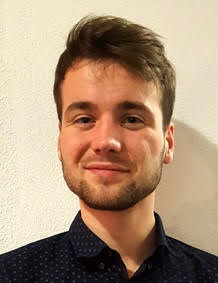
How did you get to Heyliot?
In the summer of 2020 and in the midst of looking for a company for a work-study program, I got in touch with one of the co-founders of Heyliot, Cyril Pradel. It so happened that Heyliot was looking for a profile as a data scientist, and I liked the company’s profile very much: a sensor applied in an ecological smart-city framework below any greenwashing, it was a very interesting opportunity for someone like me who is interested in the ecological issue from an engineering point of view, so love at first sight did the trick.
What is your educational background ?
I entered an engineering school in Toulouse, the INSA of Toulouse, with the initial idea of doing a biological engineering course. Once there, my heart leaned towards mathematics. So I continued in this direction and integrated a double degree between INSA Toulouse and ENSEEIHT, another engineering school in Toulouse. The double degree allows me to approach data science with artificial intelligence tools, it mixes mathematical and computer tools.
What does the job of a data scientist consist of?
All that follows is of course my own responsibility, but I think that the data scientist is halfway between the computer scientist and the mathematician. Very often, the pattern is similar: the data scientist must be interested in an interesting data, for example at Heyliot, the filling level of a container equipped with a sensor. His role is then to explain and exploit this data, for example: to transform this data to generate “meaningful” indicators (kpi), to visualize this data through graphs / diagrams (dataviz) and finally, and perhaps above all, to explain this data by other data (to elaborate a model), in particular to be able to make predictions.
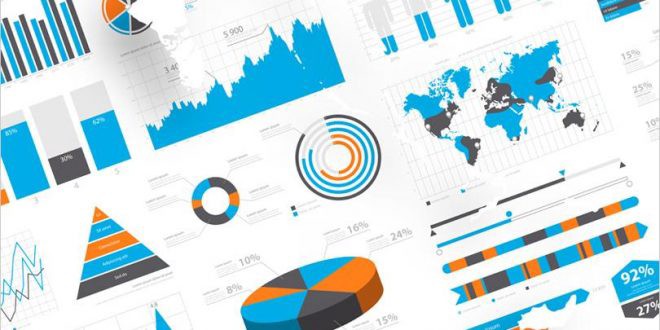
What are the main tasks?
I think that one of the mandatory steps is to “clean” the data, to denoise it. This is an essential step because data that is too noisy is difficult to explain and exploit. The second mission is the task of explaining and exploiting the data as I mentioned before. Finally, as an engineer, the data scientist also has to keep an eye on technology: on the tools used to explain and exploit the data, but also on the data sources themselves. For example, one of my roles at Heyliot is to find data that can influence the level of filling of containers: weather, traffic in a place, surrounding transportation.
What types of data do you handle?
The data that is mainly handled is the container fill level. It is simply a large table where each line contains a date and the level of filling of the container at this date. Other quantitative data such as weather data, but also potential qualitative data such as the day and month of a container measurement are added. All these data can impact the measurement and more or less explain it.
What tools do you use? And for what needs?
On the mathematical level, the tools used are mainly statistical tools: regression, modeling, classification, which can be used to exploit and explain the data. There are also other mathematical tools of analysis used in particular to denoise the data. On the computer side: the preferred tool is the Python programming language. The R programming language can be added to it, which allows easy exploration of the data. Python being a full-fledged programming language, it allows to receive the data, to manipulate it and to send back what is interesting to the developers so that they can make it available to the clients.
What advice would you give to someone who wants to do this job?
I’m not sure I’m in a good position to give advice, but I would say that being curious about math and computer science is essential. I also think that a good data scientist takes moments of reflection to address the ethics of the job. A lot of technologies are going in the direction of big data, to name a few: autonomous cars, search engines, smart-city. In my opinion, it is essential to have a well-developed ethic on these issues.
A last word?
Thank you Heyliot for sharing with me this beautiful adventure that is data science!